Many organizations have already been looking to AI to drive business growth and value — a McKinsey survey found that 63% of respondents report revenue increases from AI adoption within their company. And AI’s role is only going to become more important. In fact, it’s estimated that AI will contribute nearly $16 trillion to the global economy by 2030.
However, even though businesses recognize the importance of AI, they still have trouble implementing it. Half of all AI projects fail, and of the companies that successfully launch projects, very few of them are scaling AI across the entire organization. This happens due to several reasons: a lack of trust between the business and data science teams; AI being used in one-off tests that are cut off from the business; and not enough data scientists to meet growing demand, resulting in a lack of time to develop effective AI models (and communicate these to the rest of the company).
In our recent webinar I sat down with Manny Sevillano, the Head of Product at Virtualitics, and Aakash Indurkhya, the Co-Head of AI at Virtualitics, to tackle these challenges head-on and discussed best practices for addressing this AI gap between data scientists and business teams. Read on for five ways to help AI gain traction within your organization.
Find a Common Language
For data scientists, it can be easy to get hung up on optimization and improving accuracy, to the point that they lose sight of how AI is going to impact the bottom line. If your organization is too focused on the discovery and exploration aspects of AI, you run the risk of leaving important stakeholders behind. This is why a road map is crucial, and why your organization’s vision for AI has to be communicated to both data scientists and the business people.
“You need a common language for people to talk about that AI vision and the problems that they’re trying to solve,” said Aakash.
You can also achieve this by broadening the access to the tools that only data scientists typically use — giving the analysts and business people on your team access to easy-to-use AI tools can bring everyone onto the same page.
Make Engagement a Priority
Part of this disconnect between data and business teams stems from the simple fact that one team uses AI more than the other. “Practice makes perfect,” said Manny. “If you have a community that’s using AI on a daily basis, then you’re inherently building trust and confidence. If you go through enough feedback loops, you’re going to get meaningful value from something.”
Because data scientists are heavily involved in the day-to-day training and implementation of AI models, they can clearly see the value of AI within the organization. But that kind of engagement is siloed — in order to get business people on board, you also need to find ways to engage them in the process from the start, so that they also understand how AI can drive business value.
Invest in Visualization
Think about how you learn…well, anything. It’s always more effective to retain information when there’s a visual aid. “Most people are highly visual thinkers,” said Aakash. “If you can’t see what’s going on, then you have no ability to really trust it.”
To that end, visualization can go a long way in closing that gap between data scientists and business people in your organization. Visualization acts as the bridge between human and machine — especially as data gets more complex, you need something to help people understand what they’re looking at.
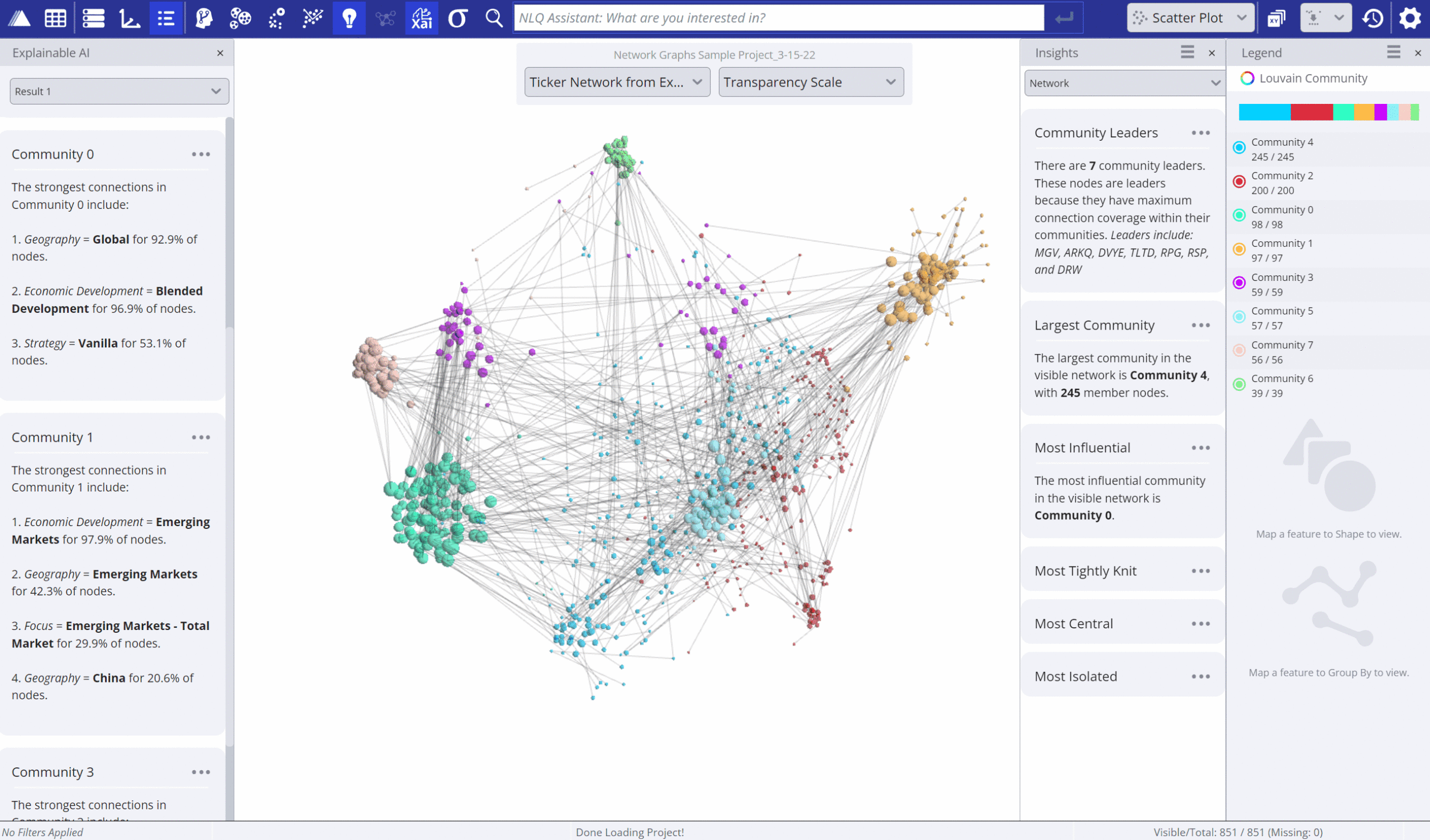
For example, in Virtualitics’ AI platform, one of the visualizations you can use is a network extractor, which takes a tabular dataset (e.g., CSVs) and transforms it into a 3D network. As a result, you can see natural clusters of different aspects of your data with similarities, as well as the relationships to other clusters. The interactivity of this visualization is key, too, as you can zoom in and out, click into any node, and automatically generate explanations, which helps tremendously with building understanding and engagement.
Focus on AI Exploration
In order to take your AI to successful production, you first need to focus on AI exploration. But exploration can be a sticking point in organizations because of how daunting and unguided it feels — there’s often so much data to sift through that it’s hard to know where to start. This opaqueness can lead to business teams downplaying the importance of AI or relegating it to one-off experiments that have no real business impact.
AI exploration is such a critical piece,” said Manuel. “Making that much easier and more accessible with visualization means people can actually use exploration as a tool to understand every step of the incremental AI process.”
You can think of it like going to a doctor’s office — if they try to prescribe a treatment but can’t explain why they’re doing it, then you’re not going to trust the doctor. Similarly, you’ll want to use visualization to provide much-needed context around AI exploration and help non-data teams understand how proper exploration can lead to more successful AI deployment.
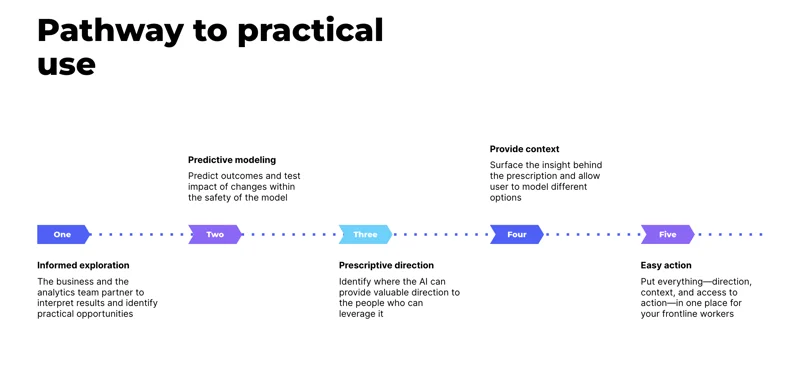
Don’t Forget the Human Element
Ultimately, people are at the core of your business, and positioning AI in a way that loops them in is the most practical path to true AI adoption. You want to make sure you don’t neglect the human element in your race to deploy AI — your teams play a crucial role in double checking the AI models and providing the strategic context necessary to ensure the AI is following your business’ overarching mission.
“Humans are still the stakeholders,” said Aakash. “AI is being built to help humans, so we should be thinking of AI as the assistant.”
By making sure people play an active role in the AI processes, you can create a more effective environment for deployment. You want someone who’s consuming data from the AI to be able to provide good feedback, which allows the AI to iterate and learn, and makes it more likely to be successful.
Make Your AI Easy and Actionable
AI doesn’t have to be a black box that nobody quite trusts. By following these best practices, you can bridge the gap between your data science and business teams, making it easier to deploy AI successfully and drive impactful business value. For even more tips, be sure to watch our on-demand webinar.