Table of Contents
Why Intelligent Exploration will save your data and AI strategy
There are big expectations of AI’s ability to contribute to business strategies – a Gartner survey found that 80% of executives expect that AI can be applied to decision-making, but only 12% of businesses have achieved enough maturity to start seeing an impact on the bottom line. Gartner reports that just 54% of AI projects are making it out of development and into production, and that’s up just 1% from 2 years ago. The promise of AI delivering value across the enterprise is stalling.
Enterprise AI is Stalling
When yet another AI project fails, fingers are typically pointed at the usual suspects—talent shortages, data quality, a lack of organizational readiness. But the truth of the failure is deeper than that. We’re trying to build a modern AI program on the backs of old tools and techniques that just can’t support the weight.
We’re capturing and storing more data than ever before, and impactful AI rests on making good use of all of that data. It’s crucial that before we start on any AI initiative that we take into account the interconnectedness of the business, assess the quality of the data, and consider enough of the right data points to ensure a usable prediction or prescription. This takes thorough data exploration.
But the way we’re exploring the data hasn’t really changed in the last 50 years. We’re trying to explore ever expanding and interwoven data sets using the same tools and techniques that we use to create dashboards and reports. In fact, in our rush to get AI into the business we may be limiting our exploration more than ever before. We’re starting off on the wrong path from the beginning and every step in the process takes us further off course.
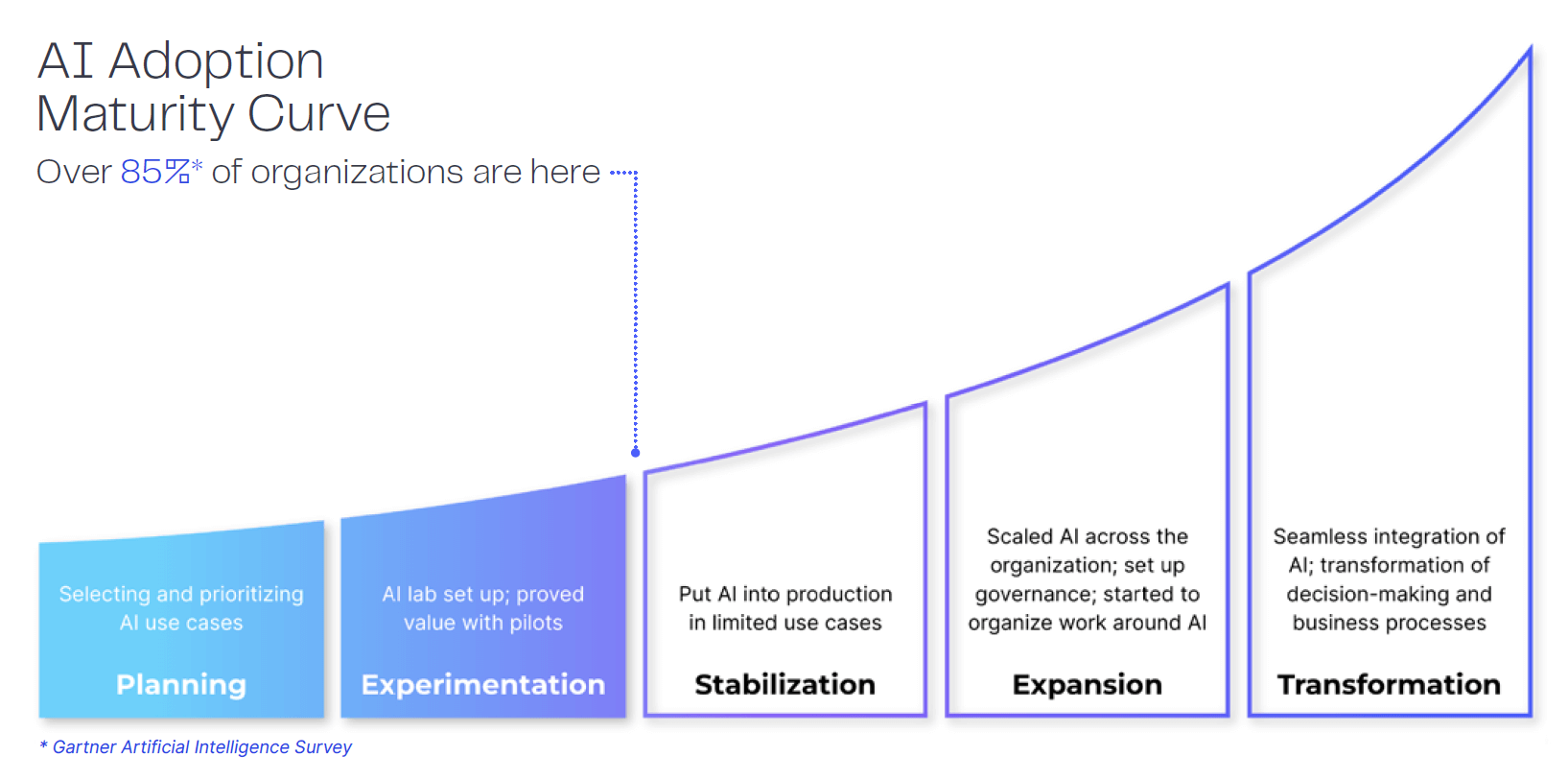
Where is data exploration going wrong?
With all of this data at our disposal we should be able to investigate problems from every angle, but we don’t. Every analysis starts with a hypothesis that is used to narrow the scope of the exploration. From the data points we include in our data set, to the questions we ask of the data, to the conclusions that we spot, the hypothesis is in the driver’s seat.
Given the limits of the analytic tools we had, it’s no surprise that analysts and data scientists have taken to using informed hypotheses to limit the scope. But hypothesis-driven exploration has always injected some risk and, as AI has amplified the potential uses of data, these risks have been amplified as well.
Hypothesis-Driven Exploration
So what is hypothesis-driven exploration?
It’s when the data is explored with a particular theory in mind:
-
Some orders keep arriving too late!Observe the problem (or opportunity)
-
We have an issue with our supply chainFormulate a hypothesis
-
Explore the supply chain dataGather only the data relevant to that hypothesis
-
Where is the weak link in our supply chain?Explore the data with that hypothesis in mind
-
Diversify the supply chain under certain circumstances. But are we targeting the right problem? Will this resolve the issue of late orders?Move forward with a project based on your results
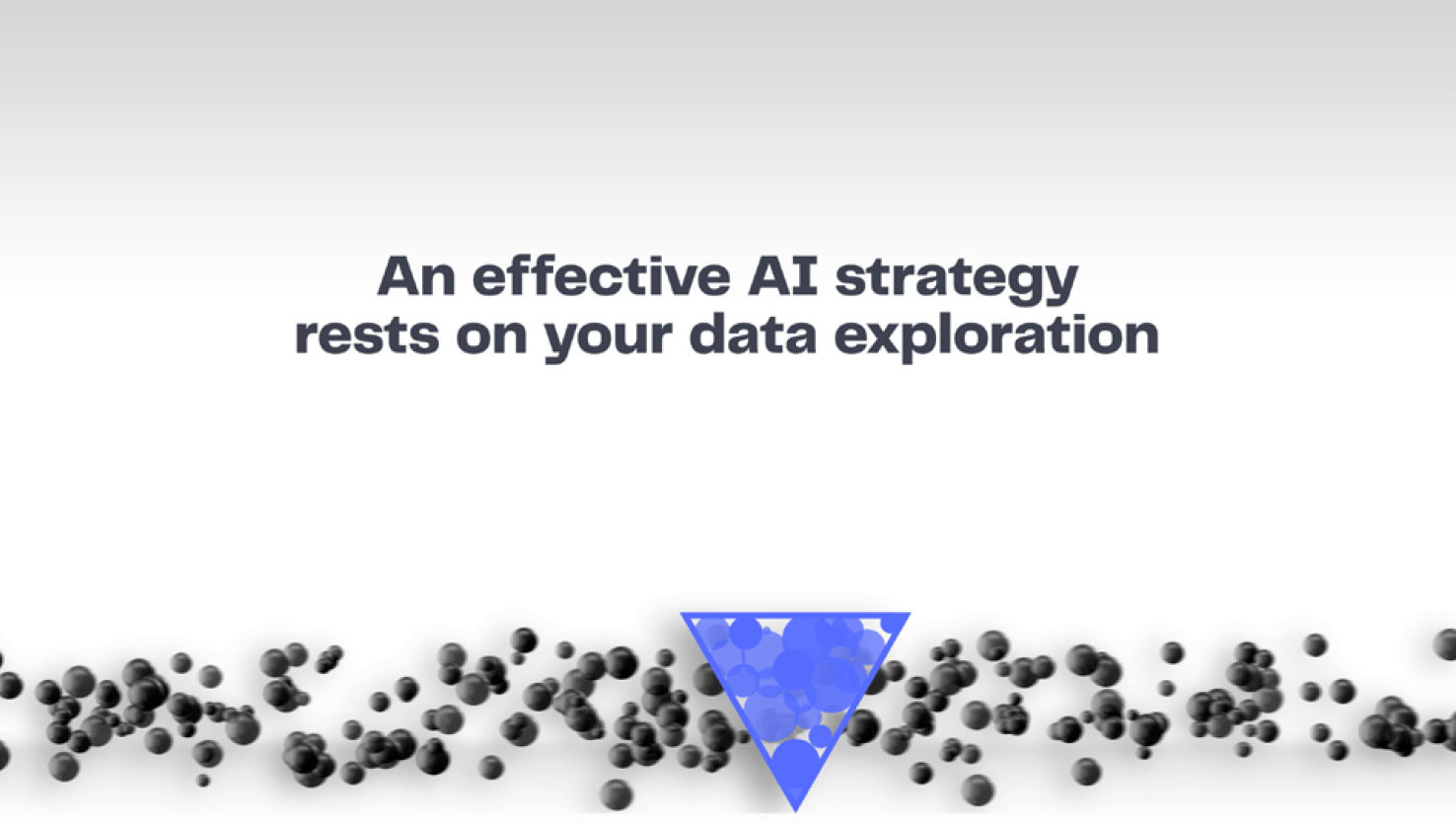
Given the time and effort data exploration takes using traditional BI tools, it’s not a surprise that data scientists and analysts have leaned on hypotheses to keep scope in check. It’s also just human nature–you have to focus your attention somewhere and it’s hard to set aside your theories to explore with an open mind. Even data scientists who develop AI algorithms to explore data will be directing their exploration using hypotheses to some degree or another.
But there are real risks to allowing a hypothesis to direct exploration:
- Missed Opportunities
You could be looking in the entirely wrong direction—what the problem is or how you should go about solving it. - Underwhelming AI or Meaningless Insights
Your exploration may yield some insight, but if it’s not insight about the real issue then any action you take will have weak or non-existent results. - Overlooked Risks
You may have left out data that pointed to big issues, or that could have left you to draw completely different conclusions. That means the real problems are left unchecked. - Biased AI
Conversely, limiting exploration could allow some data sources to have an outsized impact on the results.
Exploration should form the foundation for all of your datadriven initiatives but when it’s being done on the narrow premise of a hypothesis, everything built atop it is at risk. And when you’re planning to build AI that will automate business decisions across the enterprise the repercussions of getting it wrong are just too great.
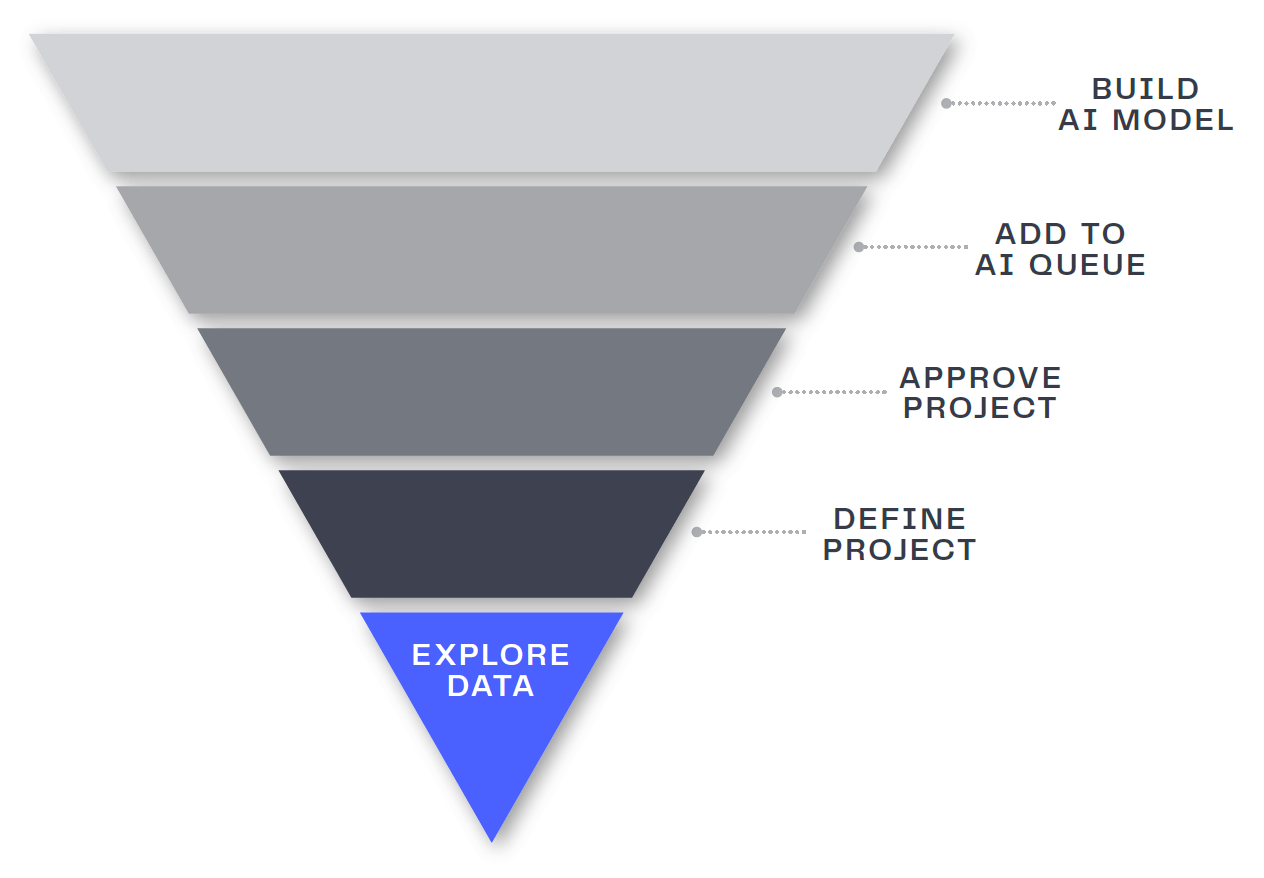
Examples of AI Gone Wrong
AI failures can lead to catastrophic losses, lawsuits, or reputational hits. Getting it right from the beginning is critical!
- Zillow’s home-buying debacle shows how hard it is to use AI to value real estate
- Payout for Estée Lauder women ‘sacked by algorithm’
- The Dutch Tax Authority Was Felled by AI—What Comes Next?
- Is Airbnb using an algorithm to ban users from the platform?
- Sexist AI: Amazon ditches recruitment tool that turned out to be anti-women
- When It Comes to Health Care, AI Has a Long Way to Go
The Talent Crisis
The lack of data scientists is hindering AI innovation and all the more reason to invest in Intelligent Exploration.
The number of data science job openings in 2022 is over 70% higher than in 20211 —and the year isn’t over yet. In short, data science teams are maxed out. They can’t afford to go down rabbit holes looking for the next big AI opportunity when they’re maxed out on basic requests.
Intelligent Exploration amplifies the power of your data science team. As author and data scientist Tobias Zwingmann expressed in a recent webinar, AI can be a powerful co-analyst. Use it to find opportunities, root out bias, and test results. You’ll get better results and put them into the hands of users while they’re still relevant.
“There’s no way to keep pace with the demand for data scientists. You gotta flip the problem and use this intelligent exploration to farm out those capabilities.”
Hear more from this conversation
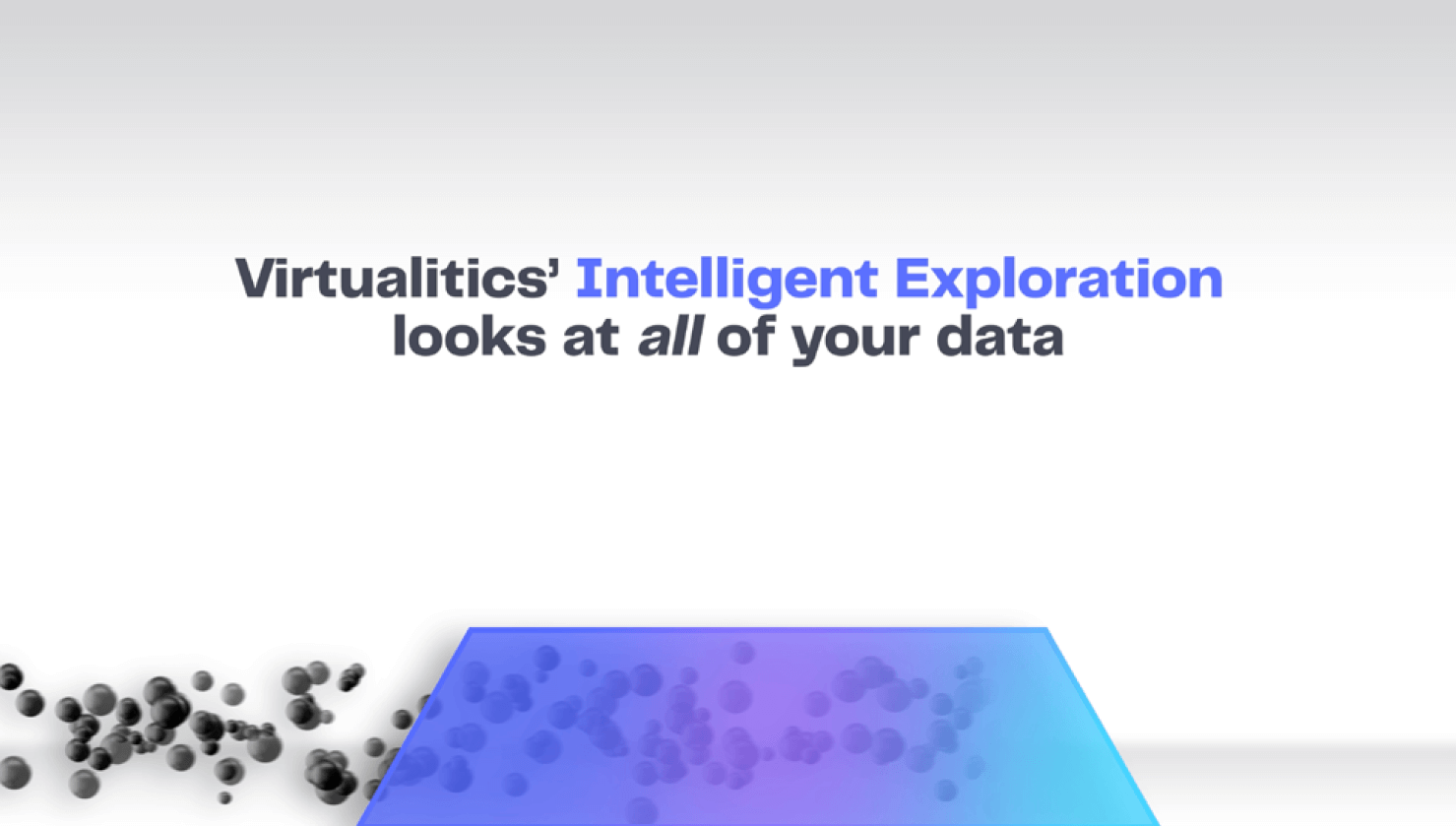
Unlock Potential with Intelligent Exploration
It’s time to change how we interrogate data. Our world is interconnected and we can’t afford to narrow the scope of our exploration. Intelligent Exploration—the use of AI to explore and visualize, and mine it for insight—must be the start of any data-driven initiative.
Intelligent Exploration flips the old hypothesis-driven exploration process entirely:
-
Some orders keep arriving too late!Observe the problem (or opportunity)
-
Gather inventory, order, and supply chain dataGather all of the relevant data
-
The biggest driver of late orders is on the order management side and changing suppliers would make no difference at all.Use Intelligent Exploration to uncover drivers behind late orders
-
Orders for certain parts need to be placed 4 weeks in advance to get on time and so inventory levels need to be flagged earlier.Pinpoint the source and the change needed
-
Develop an algorithm that tracks equipment usage and flags the projected need for the parts 4 weeks out so that the warehouse staff can order in advance to have on hand, avoiding downtime.Move forward with a project based on your unbiased results
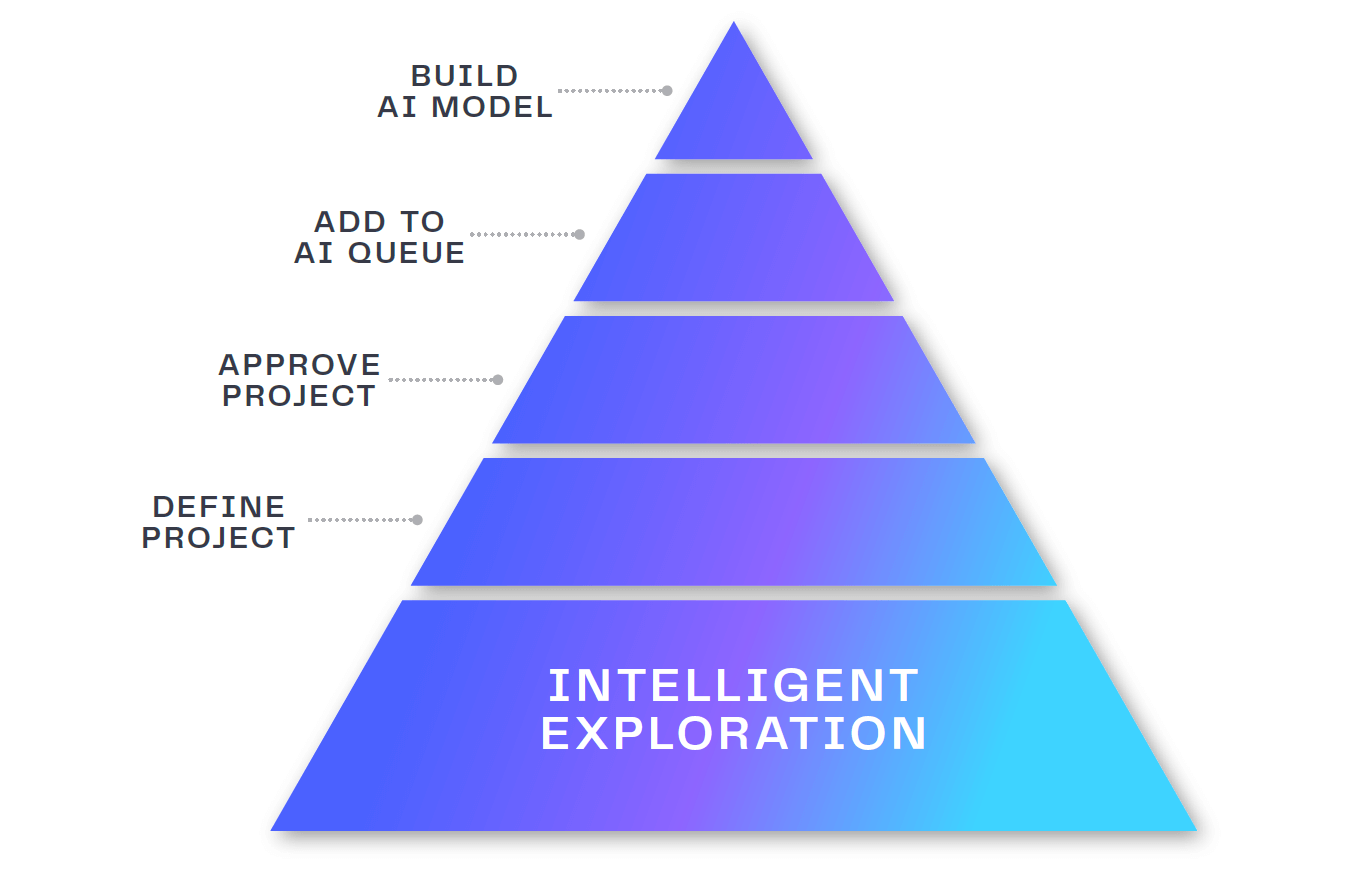
Intelligent Exploration creates a more complete picture and facilitates better decision-making because:
- It can comb through complex datasets so that there’s no need to impose our own thoughts or assumptions on what data should be included.
- Intelligent Exploration doesn’t just look at more dimensions, but it can look at the many possible relationships between those dimensions.
- It cuts through the noise of complicated data and pulls out the significant insight, so data science teams know where to focus, instead of spinning cycles trying to find the ‘Aha!’ insight.
- AI has no preconceived ideas about what trends or relationships it will find, keeping human bias out of data exploration. This means that Intelligent Exploration may find relationships that people won’t even think to look for.
- Data quality is the single most important factor in an AI model. Every model that has gone off the rails has failed because it relied on either the wrong data or weak data. Intelligent Exploration is ideal for assessing data quality effectively and efficiently.
Intelligent Exploration keeps your data science team focused on the right problems and prevents issues early on instead of when an AI app is lurching towards deployment after months of investment. With a solid data-based foundation, your business can amplify the impact of your AI programs—and your business analysts. Start strong with a data-proven baseline and you will be leading the pack in successful AI.
How Virtualitics’ Intelligent Exploration changes the game
When you explore your data using tools that restrict your breadth and depth of exploration, you’re automatically limiting the value you’ll gain from your efforts. You also miss out on opportunities for AI because you can’t see what relationships are most impactful.
Most organizations are using BI tools meant for reports and dashboards–providing snapshots into the current state–to do complex exploration intended to uncover opportunities to be predictive and prescriptive. Virtualitics is designed to do the kind of complex exploration on which organizations can build successful AI programs:
Exploring Data Today
Exploring Data with Virtualitics
- You’ve left out data that could significantly impact your conclusions or,
- One of the remaining dimensions is having an outsized effect on the insight
Your preconceived hypothesis has directed your investigation and shaped your conclusion.
- More dimensions, reducing the likelihood that significant data will get left out
- Without bias, allowing all relationships to surface, even the counter-intuitive ones
- Pulls forward the significant drivers to provide direction rooted in data, not hypothesis
- Anomaly detection provides outliers that may otherwise go unnoticed.
- Smart mapping discovers key relationships
- Network extraction constructs communities and community relationships
Stop limiting your data exploration.
Use the power of AI-Guided data exploration that will change your business.