Multitasking? Great! You can listen to this blog post by clicking above or find our podcast, Intelligent Data Exploration, on major podcast platforms.
Gartner reports that just over half of all AI projects make it into production. And of the few who do, many will go unused, either because the business doesn’t trust the models, or because they’re just not solving the right problem.
So how can you find the AI use case that will go the distance and kick off a project that will have an impact? It all starts with a good foundation.
Don’t skimp on data exploration
You can’t fix a problem you don’t understand. And you definitely can’t fix a problem if you don’t even know it exists. Data exploration is the only way to make sense of all the moving parts in your business and it’s a critical first step in any potential AI project. You need to discover where the real challenges lie if you’re to have an impact on the business. And you need to understand what is driving those challenges in order to target them effectively.
Exploration is often guided by a hypothesis–we think the challenge could be X so let’s explore to see if that’s true or not. The challenge with exploring this way is that it really limits the scope of what’s explored and has a high risk of introducing confirmation bias. Hypotheses can lead data scientists and analysts down the wrong path and away from the most meaningful discoveries. And if you’re not solving the right problem then your project is based on a false premise and you won’t be able to find and implement a solution that works.
Exploration should focus on the business challenge. Sales are down? Explore as many attributes relating to sales as possible. Want to try generating personnel schedules that anticipate demand? Explore all the attributes that impact staffing. The key is to look for all the relationships and drivers that exist, with an open mind, so that nothing gets overlooked.
If this feels daunting, don’t worry. This is where the use of AI to explore data—we call it Intelligent Exploration—can help. Traditional BI tools aren’t designed to support the breadth and depth of exploration that good AI demands as a foundation. But when you leverage Intelligent Exploration, you can start to surface the most impactful opportunities for AI.
Identify your opportunities
Very few business challenges are straightforward so your exploration will probably identify a few challenges and, for each of those challenges, a few contributing factors. The next step is to refine your results to identify which challenges are significant and worth pursuing.
Start by consulting your business stakeholders and reviewing the results of your exploration. Their knowledge of the business can help inform your understanding of your findings and together you can translate your findings into potential use cases. Involving the business stakeholders early also helps to ensure they understand how AI projects develop and what data will ultimately be used to drive the model.
Making sure that your business partners really understand the insight you’ve uncovered, particularly if it involves multiple, interconnected relationships, can be challenging but it is possible:
- Structure and present your discovered insight with a storytelling narrative.
- Set the scene (the high-level challenge you were exploring)
- Point out the early areas of insight that were surfaced by the AI and caught your attention, then what you chose to look at next as a result
- Describe the big ‘ah-ha’ insights that came into focus as potential use cases
- Use plain language wherever possible. It’s so easy to slip into the jargon that we use every day but unfamiliar terms can be distracting and make them feel like what you’re showing is beyond their comprehension.
- Be very thoughtful about which visualizations you use to illustrate your findings, particularly if you need to highlight the interplay between attributes.
For example, if you want to show the interplay between 3 attributes, showing three 2×2 plots will not get that information across in a consumable fashion but a true 3D visualization of a 3×3 will. - If your toolset allows it, leave the analysis with the business leaders to consume and explore on their own. Ideally, you’ll be able to annotate it, calling attention to the areas of interest. Doing this provides the team more time to digest what you’ve told them, and to “kick the tires” of the results.
Refine use cases and create a ranked list
Together with your business stakeholders, plot the use cases by feasibility and business value. Create and prioritize the list of possible problems to solve so that the most important challenges are addressed first.
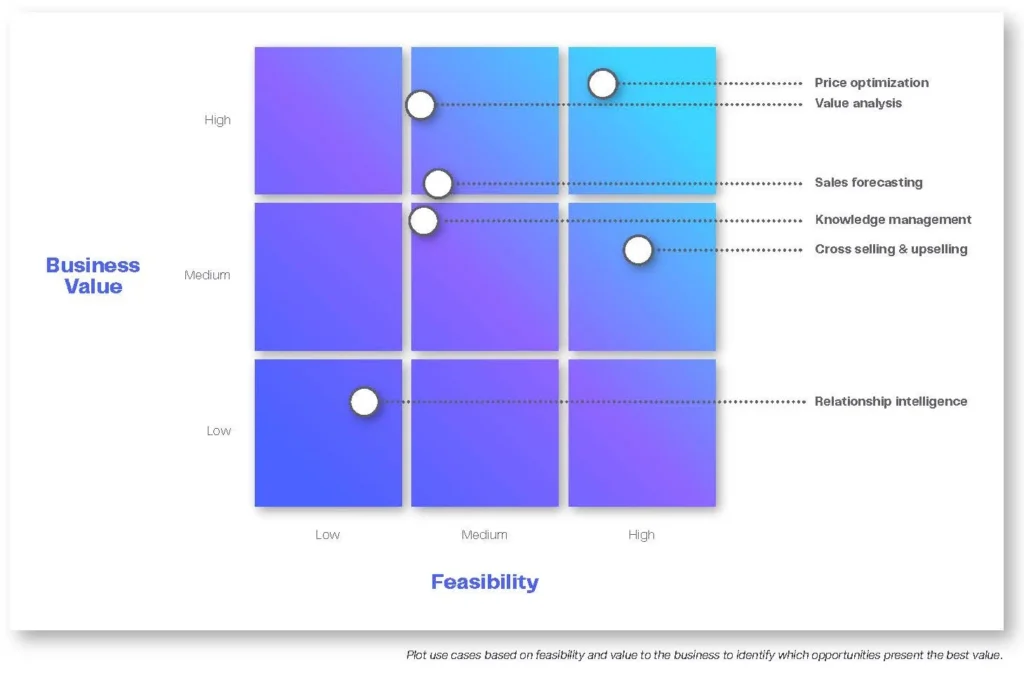
When determining feasibility consider the following:
- Should the use case be tackled with an AI model or could it be solved some other way? Just because you have a hammer, doesn’t mean that everything is a nail. Other solutions could be a process change, a system modification, or better or more timely analytics.
- Determine the cost or impact of not addressing the problem. This will help determine impact, mobilize the business to embrace the solution, and provide you with key metrics to measure success down the road.
- Can you put this model into production, support it, and foster the organizational change required to leverage it? A complex model that can be seamlessly integrated into a current workflow may be more feasible than a simple model that requires a big change to established processes.
- Fairly assess your data sources. If the data you have is weak, it won’t matter how good your report or AI model is; it won’t be usable. In fact, it could do harm.
Select The Right Use Case
Armed with your carefully plotted use cases, the result of thorough exploration and consultation with the business, you, your team, and your business leadership will be able to select the right AI use case to move forward with.
To learn more about how to successfully create the next-generation AI strategy that leadership is asking for, download our eBook “Building a sustainable AI strategy from the ground up”.